Nowadays, one of the main strategic goal of every retail business is to build a strong relationship with their customers and accelerate growth. To achieve this goal, many businesses are trying to change the way they are working and making decisions, but they are not using data they have about their customers. In today’s business world the only way to be a leader is to shift your business to data driven business.
Machine learning helps and supports transformation to data driven retail business and offers various opportunities. An understanding of the right data sources can drive new decisions and approaches. Core incentive for businesses to use ML models is a need to understand the customer’s preference and to create new personalized experiences.
86% of consumers say that personalization plays an important role in their buying decisions. Millennials are especially interested in purchasing online, and assume they will receive personalized suggestions.
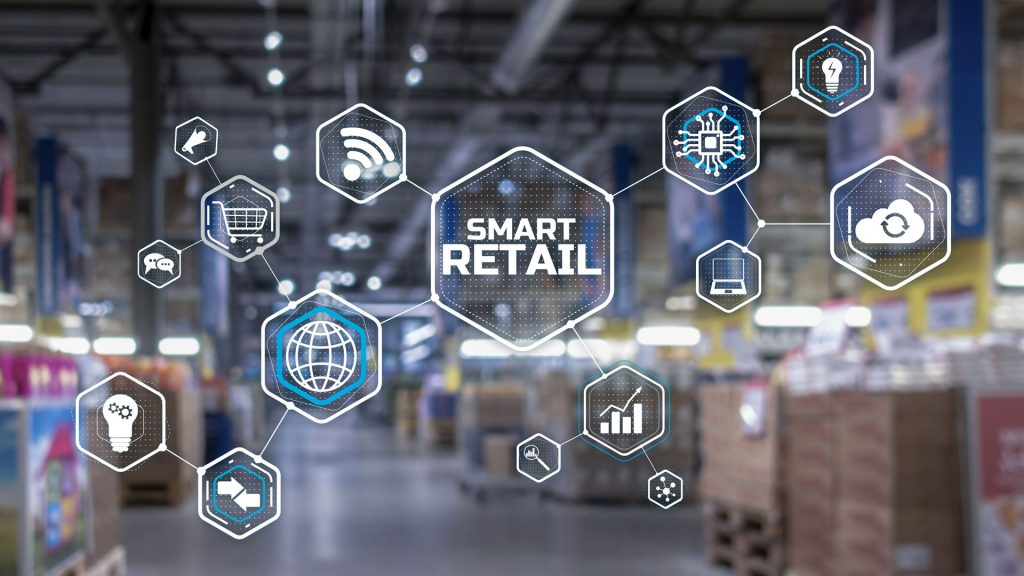
Retail businesses have a competitive advantage when it comes to data because of the quantity and quality of the data they hold. Large market players in retail industry such as Amazon, Walmart, Alibaba and Starbucks recognized the value of ML and create a lot of different use cases that utilize ML models to scale their businesses.
There are many applications of Machine learning models in the retail industry, but here are top 5.
Top 5 Machine Learning models retail businesses can use for:
- Customer profiling and personalization
Customers have different personalities and purchase with different objectives. Machine learning models help retailers to create an ideal customer profile and understand their purchase preferences and intentions. Business can easily and in the right way target customers with personalized offers, because recommender systems help retailers to offer customers products that match with their preferences and behavior. One of the key reasons why every retail business has to use ML models such as Customer segmentation is because segment characteristics indicate to the retailers how to approach which customer profile, which customers should be activated through special offers, and which should be motivated to become premium with appropriate conveniences.
- Building customer loyalty database
For retail business one of the main pain points such as creating loyal and long-lasting customer relationships and build customer loyalty data base can be overcome by using predictive analytics. For retail business, to build and retain customer loyalty database it is necessity to take care of every customer. Churn models could recognize customers whose activity started to decline. The goal of this model is the early identification of customers who are likely to stop purchasing products from the certain retailer, so the retailer can react and retain them. Why is this important? 59 percent of US respondents to the survey by PricewaterhouseCoopers (PwC) noted that they will say goodbye to a brand after several bad experiences, and 17 percent of them after just one bad experience. Nevertheless, keeping existing customers is five times cheaper than the cost of attaining new ones. For this reason, marketing executives often find themselves trying to estimate the likelihood of customer churn and finding the necessary actions to minimize the churn rate.
- Recognizing fraud
Machine learning models learn on customers’ previous behavior, they recognize its patterns and anomalies. Therefore, if it happens that a transaction is very different from something typical for specific customer, it could be identified as risky. Speaking of having an account on eCommerce website, what is also considered as fraud detection is identity theft. Using the models that are able to find correlations in various data sources and point out warnings about specific customers or transactions, could be of utmost importance for companies. On the one hand, it is beneficial for the company and on the other it has many advantages for end-customers.
- Inventory optimization
When it comes to retail, some of the major challenges are the amounts of inventory held in warehouses and choosing the right locations for them. Having the ML model that optimizes amounts of inventory enable companies to always have enough products when they are needed. Not being ready to answer demand in the right time, can represent significant cost for companies – longer customer’s waiting and additional cost of product shipping. With appropriate locations of warehouses and enough products, companies could provide efficient services and maintain customer satisfaction.
- Image recognition
Advanced modeling concepts empower retailers to enhance the way how they inform their customers about their offerings. For instance, using image recognition for advanced search can give customers possibility to quickly find what they are looking for. Even if a product of their interest is not there, the algorithm could show them the most similar one.
Conclusion
Machine learning models have found wide application in various industries, but the retail industry has been particularly changed. Machine learning covers various challenges of retailer’s everyday activities and helps them to have data driven business. With right ML models retail business can create customer segments which provides a better understanding of customer behavior and preferences. Moreover, the customer satisfaction and their loyalty are among the essential points that ML brings.
Authors:
Authors:
Get to know
your customers
better.
FAQ | Finance | Banking | Telecommunications | Retail | Why Selecta | How it Works