Six ways Machine Learning models can help banks
Machine learning is a prerequisite for an intelligent system that supports the banking sector. Without this, there is no possibility of data-based predictions that can create new business opportunities for individual institutions. The entire industry is already taking advantage of this technology in order to be able improving products and adapting services to all customers. How machine learning models can be used for different purposes and the value they create is diverse. Some of the main values that stand out are:
1. Understanding customer profiles
2. Personalized content for customers
3. Process automation
4. Maintaining the customer base and customer satisfaction
5. Financial consulting
6. Early signaling of company risk
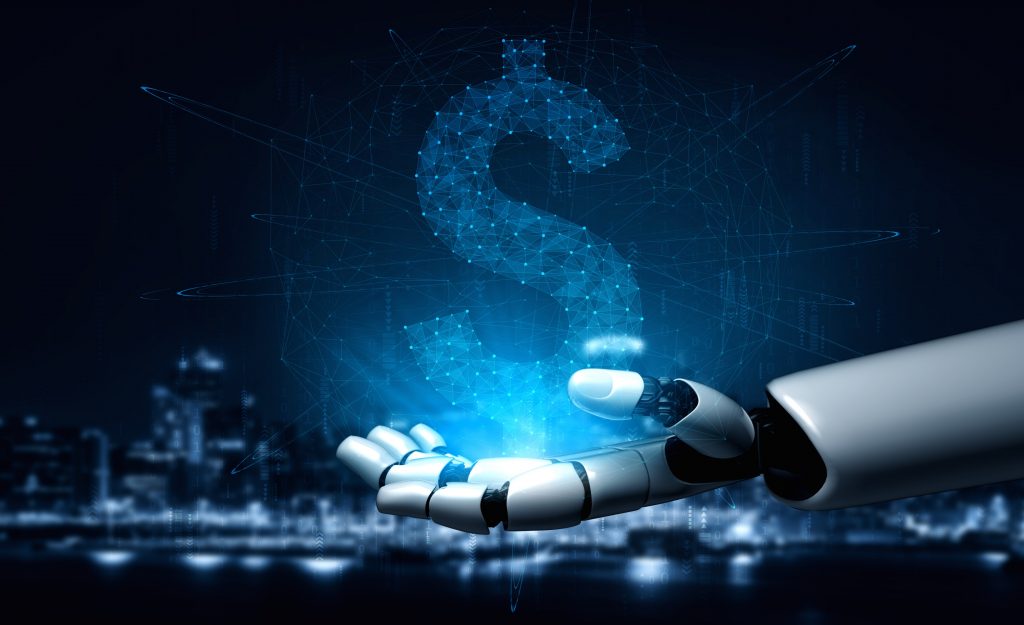
Understanding customer profiles
By using different models of machine learning, bank customers can be segmented so that different profiles can be distinguished. Each of them has its characteristics and needs. Knowing which segment a particular customer belongs to, allows the bank to adjust its approach and more precisely determines the target group for the offers of its products and campaigns. Approaches to segmentation are different. The differences in the segments are reflected in the payment parameters, as well as the way money is spent. Some of the segments are more prone to more frequent and smaller purchases, while on the other hand there are segments that are more prone to larger purchases that they make less often.
Personalized content for customers
Nowadays, the personalization of the customer experience plays a big role in the purchase. 80% of people are more likely to be clients of companies who work on personalizing the experience. Machine learning models based on the customer’s behavior towards the bank calculate the customer’s tendency to use a particular product or service. Based on this information, banks can not only conduct more efficient campaigns but can also optimize their portfolio according to the identified needs of customers. Another type of personalization is the establishment of communication through customers’ preferred communication channels.
Process automation
Process automation within the bank is established by starting them when certain model outputs are obtained. Processes include, for example, sending campaigns or opening CRM retention processes to dissatisfied customers. By monitoring changes in real-time and the daily launch of the model, it is possible to monitor each customer’s action and its impact on the models. If the customer files a complaint, it may affect the output of the churn model. When the churn value for this customer changes, the bank can define that this starts the CRM retention process and automatically starts resolving the problem.
Maintaining the customer base and customer satisfaction
The importance of maintaining customer satisfaction is financially reflected in the amount of the cost of acquiring new customers, according to retaining existing ones, as well as the additional income that loyal customers generate. For this reason, banks design numerous loyalty programs in order to maintain customer satisfaction. For 6 years in a row, Tangerine Bank of Canada has been the preferred Canadian bank, thanks to its loyalty program with special credit card conditions (2% money-back credit card program). In this way, it has set the standard for all modern financial institutions.
Financial consulting of customers
With Machine learning models based on the bank’s customer consumption, ie. transactions, it is possible to automatically classify customer transactions, predict overspending, or suggest an optimal savings plan for a specific goal set by customers. An excellent example of the use of artificial intelligence for the purposes described is the Spanish multinational financial services company BBVA. By personalizing the customer experience while using the bank’s application or by communicating with the bank’s virtual assistant (chatbot), customers receive valuable information about their spending and advice on how to improve it if they wish. The models described in this way contribute not only to the bank’s internal processes but also to the bank’s customers. The value is two-way.
Early signaling of company risk
In addition to the stated advantages of machine learning that apply to individuals, some advantages are also aimed at the bank’s clients who are companies. By using the model for early risk signaling of companies, the bank can detect a risky client in time and apply preventive measures, to reduce the impact on its business. The main advantages of the model are time and money, which the bank can save thanks to the information it obtains using artificial intelligence.
Get to know
your customers
better.
FAQ | Finance | Banking | Telecommunications | Retail | Why Selecta | How it Works