Analytical Module
and Big Data
Did you know that 84% business think that AI will enable them to obtain competitive advantage?
A company that manages to react to customer needs in real time and offer the right products/services on the preferred channel will become the customer’s first choice, and thus emerge as the market leader.
Those that fail to embrace Artificial Intelligence (AI) and the benefits that come from unlocking data, on the other hand, risk being left behind by those that do.
By observing and listening to customer needs, companies can maximize their retention rate, attract new customers, and accelerate into the digital future.
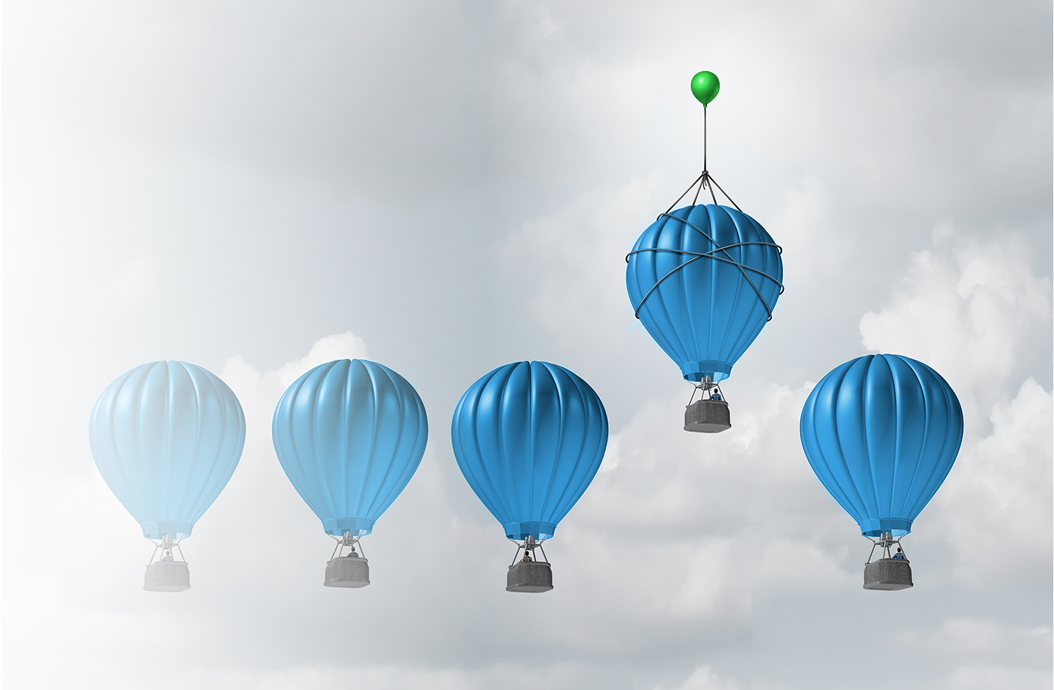
Did you know that 84% business think that AI will enable them to obtain competitive advantage?
A company that manages to react to customer needs in real time and offer the right products/services on the preferred channel will become the customer’s first choice, and thus emerge as the market leader.
Those that fail to embrace Artificial Intelligence (AI) and the benefits that come from unlocking data, on the other hand, risk being left behind by those that do.
By observing and listening to customer needs, companies can maximize their retention rate, attract new customers, and accelerate into the digital future.
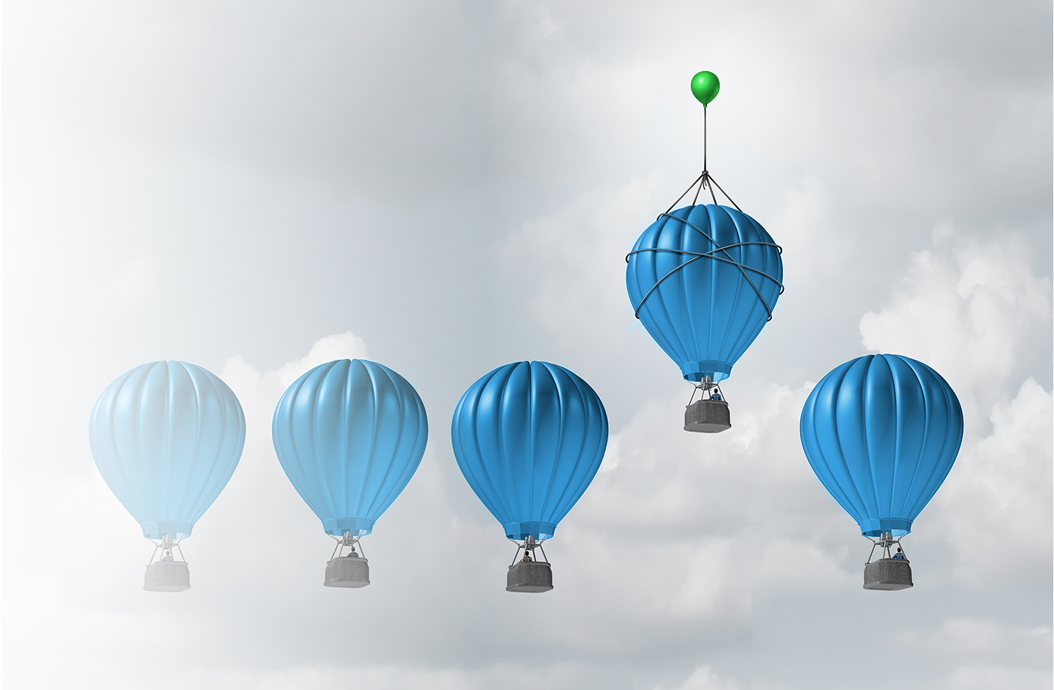
Process flow
DDATA COLLECTION AND PREPROCESSING
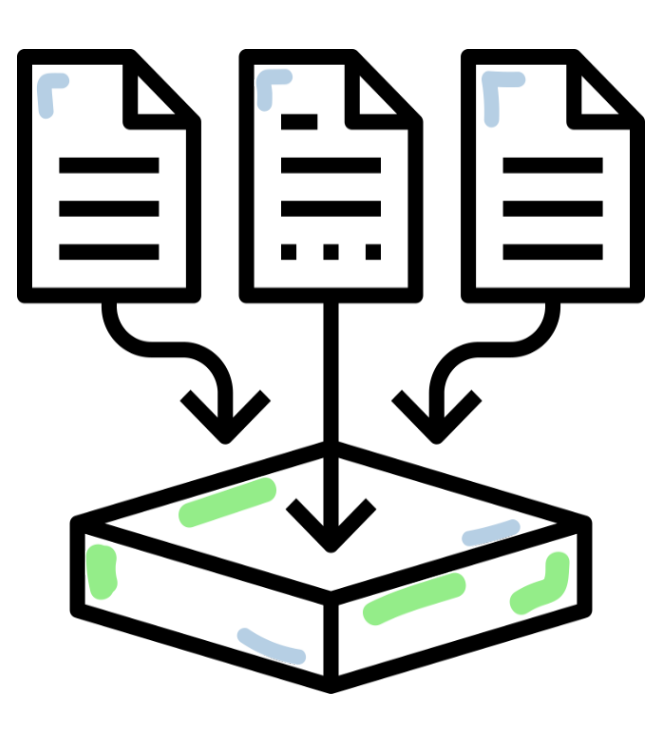
Leveraging immense amount
of collected data for gaining
essential customers insights
ML MODELS
DEVELOPMENT
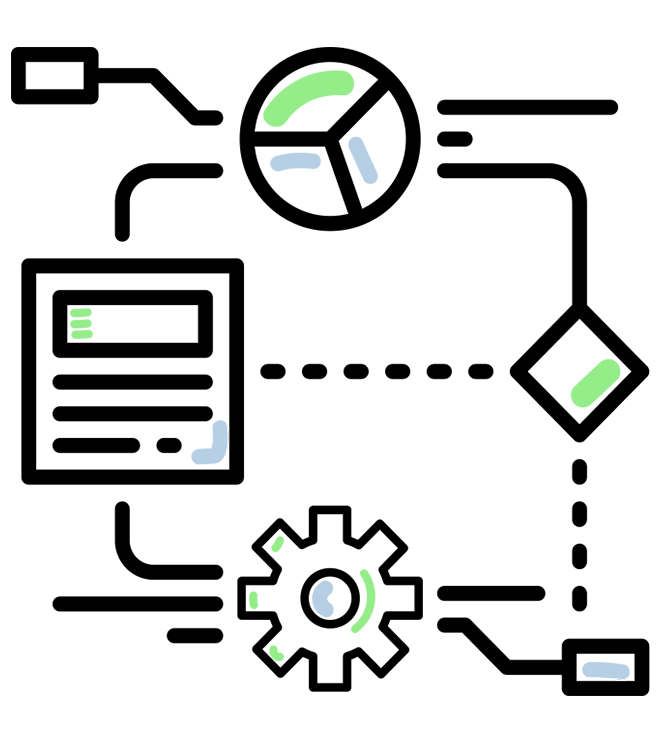
Predicting customer behavior
by using AI prediction
and high-performance ML algorithms
ENHANCING
CUSTOMER RELATIONS
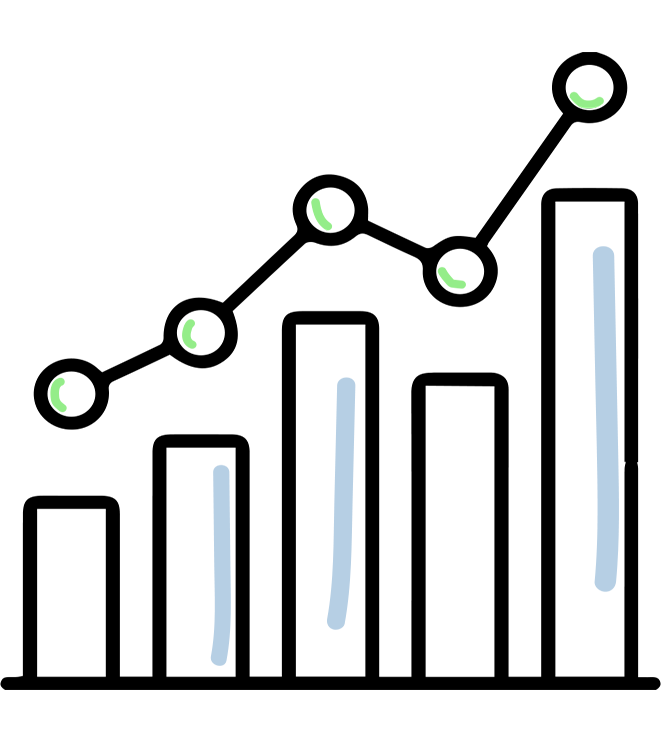
Creating personalized experienced
and strong relations based
on deep customer understanding
Advanced analytics
Machine learning models enable companies to personalize approach towards their customers, understand their needs and to create and successfully optimize customer journeys. Analytical module consists of the wide range of ML models, which is devided in three groups. These are: Core models, Spending models and Event-related models.
Core models
Enhanced campaigning process, increased conversion rate and customer satisfaction due to personalization as well as ensuring understanding of customer behavior and their preferences are key benefits of Next Best Offer and Customer segmentation models. With Churn prediction models the goal is early identification of customers who are likely to leave. Making decisions about the customers in which the company should invest the most and tracking effects of conducted campaigns are supported by Customer Lifetime Value model.
Next Best Offer
RFM Customer Segmentation
Churn prediction models
Customer Lifetime Value model
Spending models
Pointing out customers’ habits as well as creating personalized offers and bonuses based on customer behavior is supported by Spending models. Additionally, Transaction Categorization allows banks to track and monitor changes in customer spending. Advanced Segmentation model enables tracking migration between the segments, as well as fostering customer loyalty and satisfaction. Overspending detection and Saving Plan model help customers to be aware of their spending behavior and manage their finances better.
Transaction Categorization
Advanced Segmentation
Smart Finance Planning models
Premium Detection model
Event-related Models
Event models enable identifying customers’ tendencies for important events that a company has created. In this way, numerous customers who almost fulfill the rules would not be missed. Besides tendencies, event prediction models predict how certainly the customer will complete defined events. In a nutshell, the company is aware of customers who satisfy the events completely and also the ones who are very inclined to make them.
Event Tendency
Prediction models
Next Best Offer
Model for product and service recommendation, NextBestOffer, calculates customer tendency to make a purchase, or inclination to use a certain product or service that the bank provides. This predictive model makes decisions based on customer’s actions and behavior toward the company. The model uses available data gathered from various sources from previous months and makes predictions for the following period. NBO model empowers companies to recognize the clients who will purchase their products or services with 85% reliability.
The essential values of the Next Best Offer models are:
Enhanced campaigning process, increased conversion rate and Increased customer satisfaction due to personalization.
RFM Customer segmentation models
Customer segmentation provides a better understanding of customer behavior, as well as tracking movement of the customers between segments. The established segments should enable better customer insight and response to their needs and desires. Migration between segments can provide information about migration frequency, the stability of segments, and other important factors. Segment characteristics indicate to company how to approach which customer profile, which customers should be activated through special offers, and which should be motivated to become premium with appropriate conveniences.
There are six types of segmentation that are used. All of them rely on three key payment parameters: recency, frequency & monetary.
Churn prediction models
Churn model will recognize clients who intend to stop using a certain product or service. The goal of this model is early identification of customers who are likely to leave the company, so the company can react and retain them. This information could be available through 360 customer profile of Operational part of Selecta CRM solution, or could be used for sending campaigns to the specific group of clients. The aim of this prediction model is to improve the retention rate.
Data sources for this type of model include transaction data, channel data (logging details, call center calls, etc.), chatbot conversations, products used, blacklist data, complaints, internal client classification, and more.
Customer Lifetime Value model
Customer Lifetime Value predicts the value of a customer for a one-year period. It models customers’ purchasing behavior in order to predict what their future value will be. CLV model makes predictions based on a wide variety of data. Data sources include customer demographics, product-related data, customer income, customer spending and payment channels.
Intelligent Transaction Categorization
Advanced Segmentation model
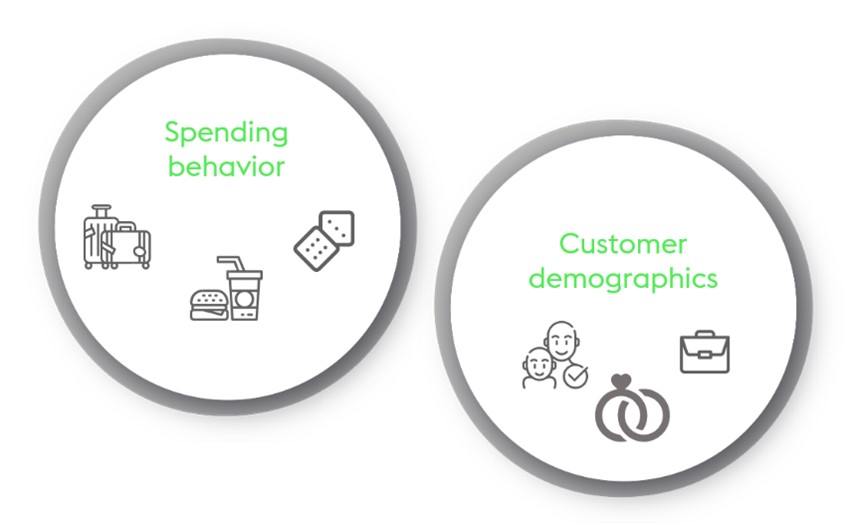
Smart Financial Planning
They include Spending tendency models, Overspending Tendency and Prediction, Saving Plan Recommendation
Spending tendency models
Spending Tendency model is characteristic of the banking industry and calculates customer tendencies for spending on different categories based on its previous behavior. It enables identification of customer hobbies and habits. Furthermore, the Bank can provide all spending information to the customer who asks for it (over ebanking, mBanking or Chatbot).
Overspending Tendency and Prediction
Overspending Detection models provide identification of customers who have high overspending tendencies or will overspend in the future. Overspending detection has several use cases. For instance, sending personalized offers or notifications.
Saving Plan Recommendation
Saving models are making recommendations to customers, which represent saving plans for their future goals (trip or something else).
Event signaling module
Event signaling begins with the identification of all events related to the user. Includes life events, work events, and events related to the company’s products. All events are signal triggers that allow the company to create a highly personalized approach, perform precise targeting and respond on time.
Each customer’s action represents trigger for signaling in real-time that enables companies to create a highly personalized approach, conduct lightweight and precise targeting and react on time. Behind real-time monitoring of an immense number of events stands the advanced Complex Event Processing Module. This Module allows the company to create aggregated events, which are signaled when occur. The company’s employees could set rules according to their needs. Furthermore, event-related models provide significant flexibility in identification of the right customers.
Early Warning System model
Early Warning System model predicts the status of the bank’s customer for the next 6 months, based on transactions and other types of customer data. This implies that a potential or existing problem in their business can be identified, so it has minimum influence on the bank. The result of the model represents one of three defined signals (red, yellow and green) which also provide descriptions about the reason why they appeared. In this way, the bank will know that the problem exists and where to look for in further communication with the customer. All statistics and visuals will be available over a user-friendly interface, suitable for its users. The model with 80% reliability identifies which clients will become risky and which will not. This leads us to the conclusion that in 8 of 10 cases bank will know is the customer risky one or not.
The application includes advanced tools and many filtering options, most importantly, all essential information is at one place. The application user is also provided with different statistics related to signals, historical records, trends, etc.
Analytical 360° customer view
Analytical 360° is user-friendly application that enables the companies to:
- Track technical metrics and performances of the ML models
- Have an overview of the general and individual customer data
- Make data-driven business decisions
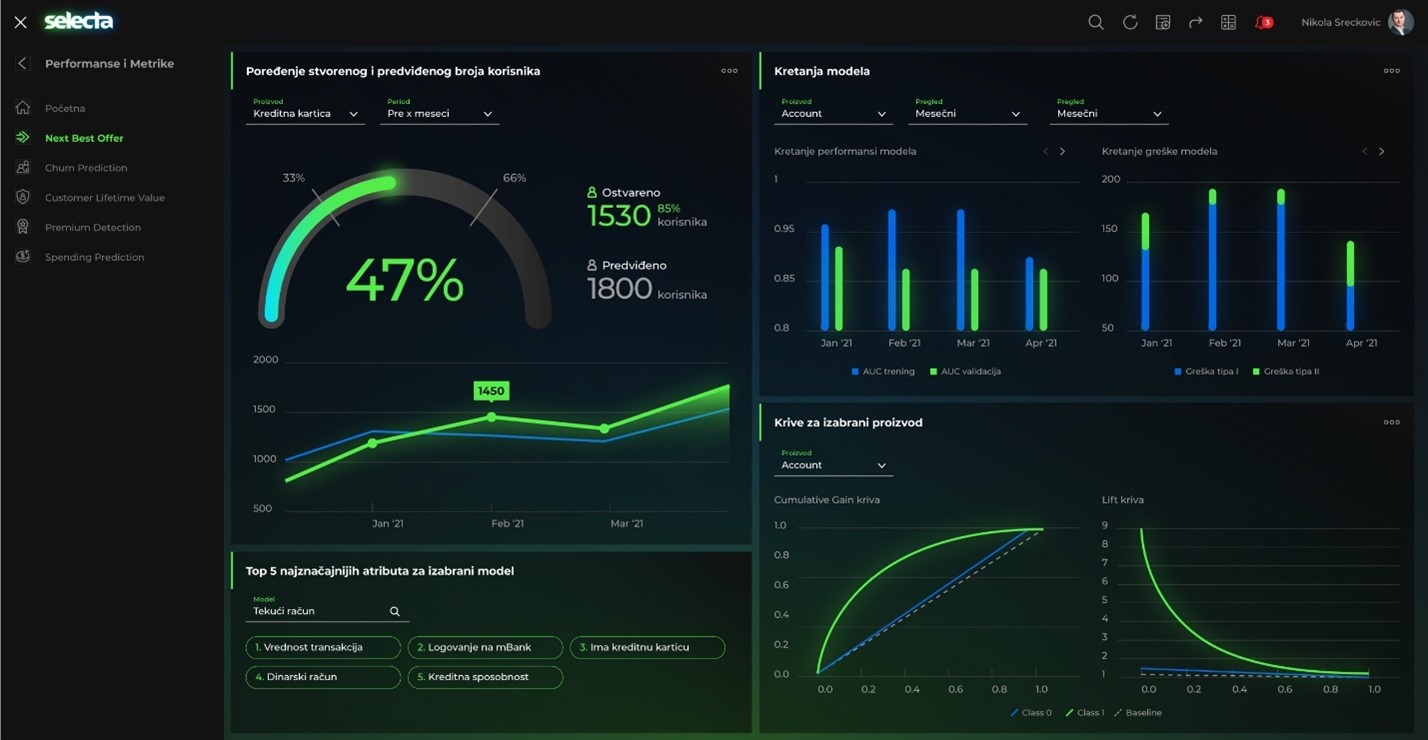
Analytical 360° customer view
Analytical 360° is user-friendly application that enables the companies to:
- Track technical metrics and performances of the ML models
- Have an overview of the general and individual customer data
- Make data-driven business decisions
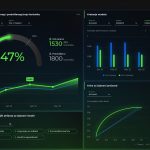
Selecta’s success
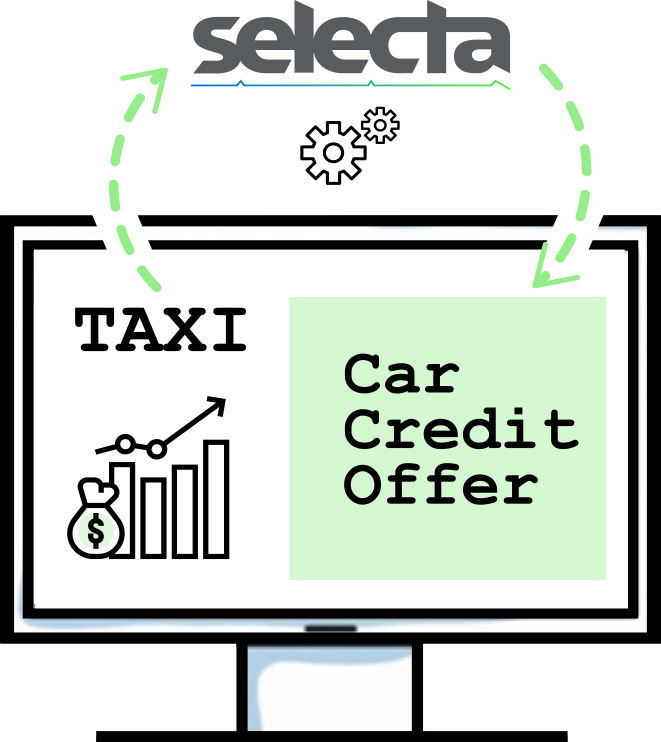
NBO model empowers
companies to recognize the
clients who will purchase
their products or services
with 85% reliability.
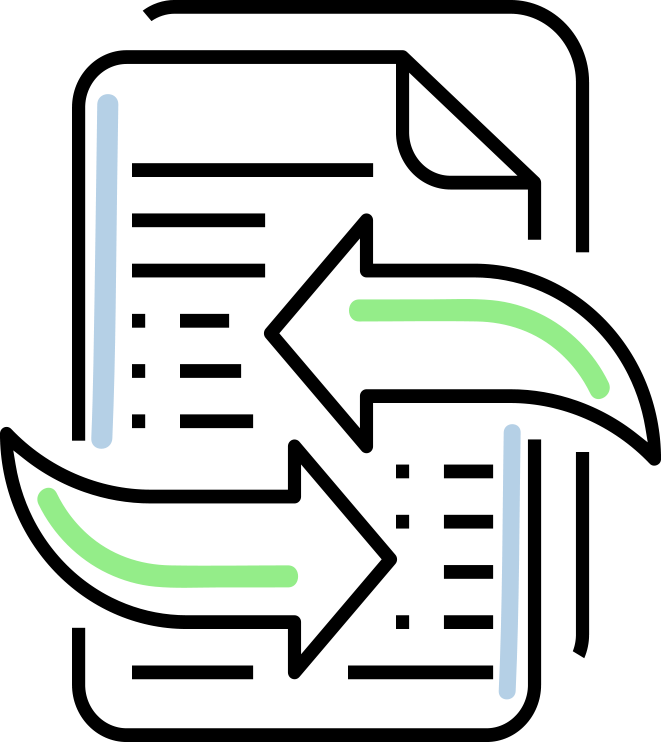
Reliability of our EWS model
is about 80%
in predicting
default status of
the bank’s client
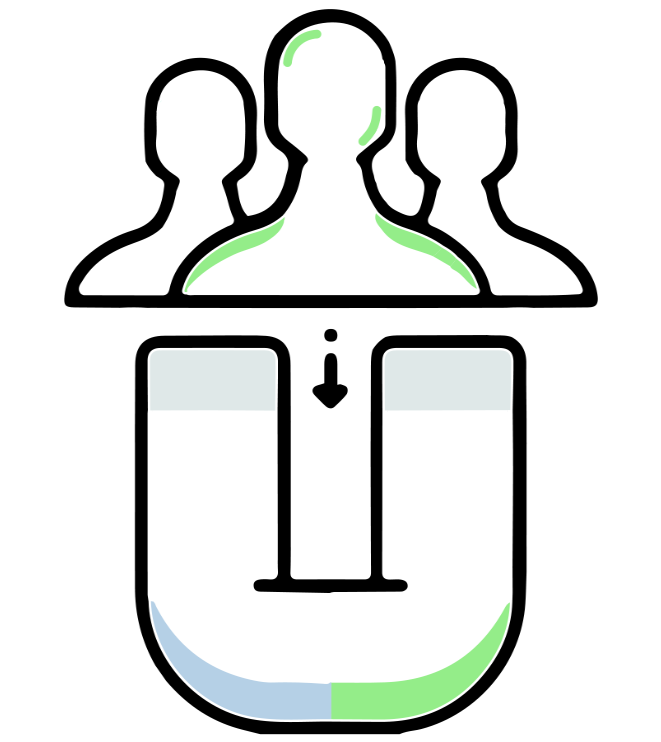
Using machine learning
powers companies with an
immense capability to
identify precisely potential
premium clients.
Selecta’s success
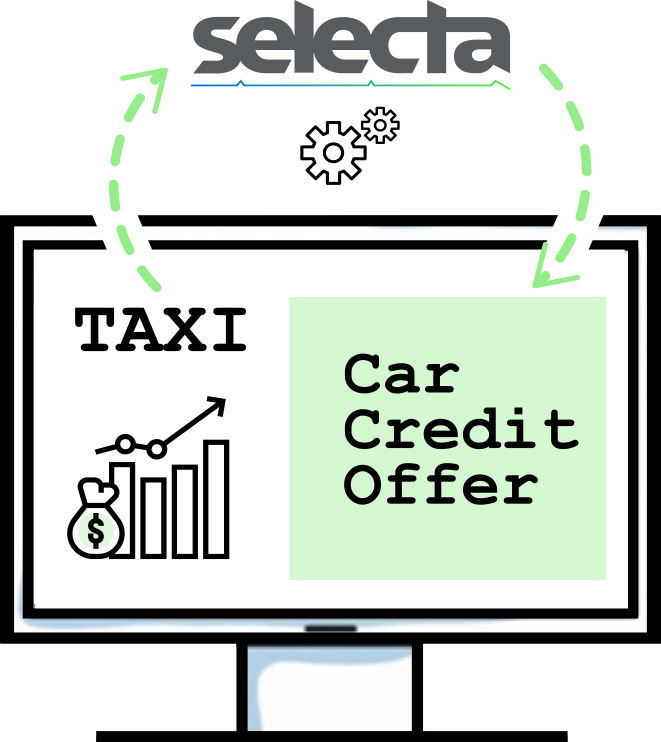
NBO model empowers
companies to recognize the
clients who will purchase
their products or services
with 85% reliability.
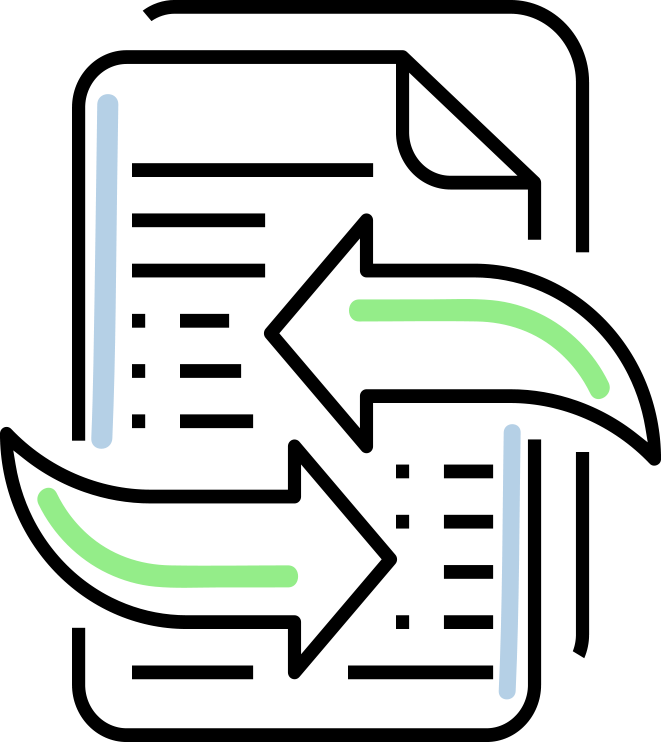
Reliability of our EWS model
is about 80%
in predicting
default status of
the bank’s client
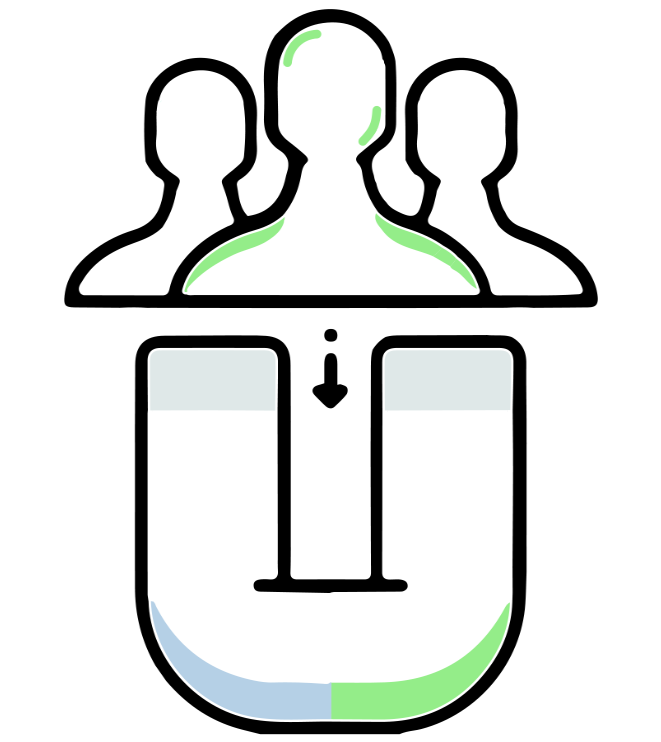
Using machine learning
powers companies with an
immense capability to
identify precisely potential
premium clients.
From unstructured data
to a satisfied customer
Selecta is collecting data from all possible sources, whether it is a website visit, a transaction in eBanking, or logs into a personal account in the app or website, a conversation with operators from Call center, a chat with a company chatbot, or a simple visit to a shop or branch office. This data is processed and represents inputs for machine learning models. Each of these models has outputs which represent benefit to the company – how to increase sales, retain existing customers, and increase customer satisfaction. The Next Best Offer model provides a personalized offer for your customers, Segmentation model provides better customer profiling, while Customer Lifetime Value predicts how much a company will have a value from each customer.
From unstructured data to a satisfied customer
Selecta is collecting data from all possible sources, whether it is a website visit, a transaction in eBanking, or logs into a personal account in the app or website, a conversation with operators from Call center, a chat with a company chatbot, or a simple visit to a shop or branch office. This data is processed and represents inputs for machine learning models. Each of these models has outputs which represent benefit to the company – how to increase sales, retain existing customers, and increase customer satisfaction. The Next Best Offer model provides a personalized offer for your customers, Segmentation model provides better customer profiling, while Customer Lifetime Value predicts how much a company will have a value from each customer.
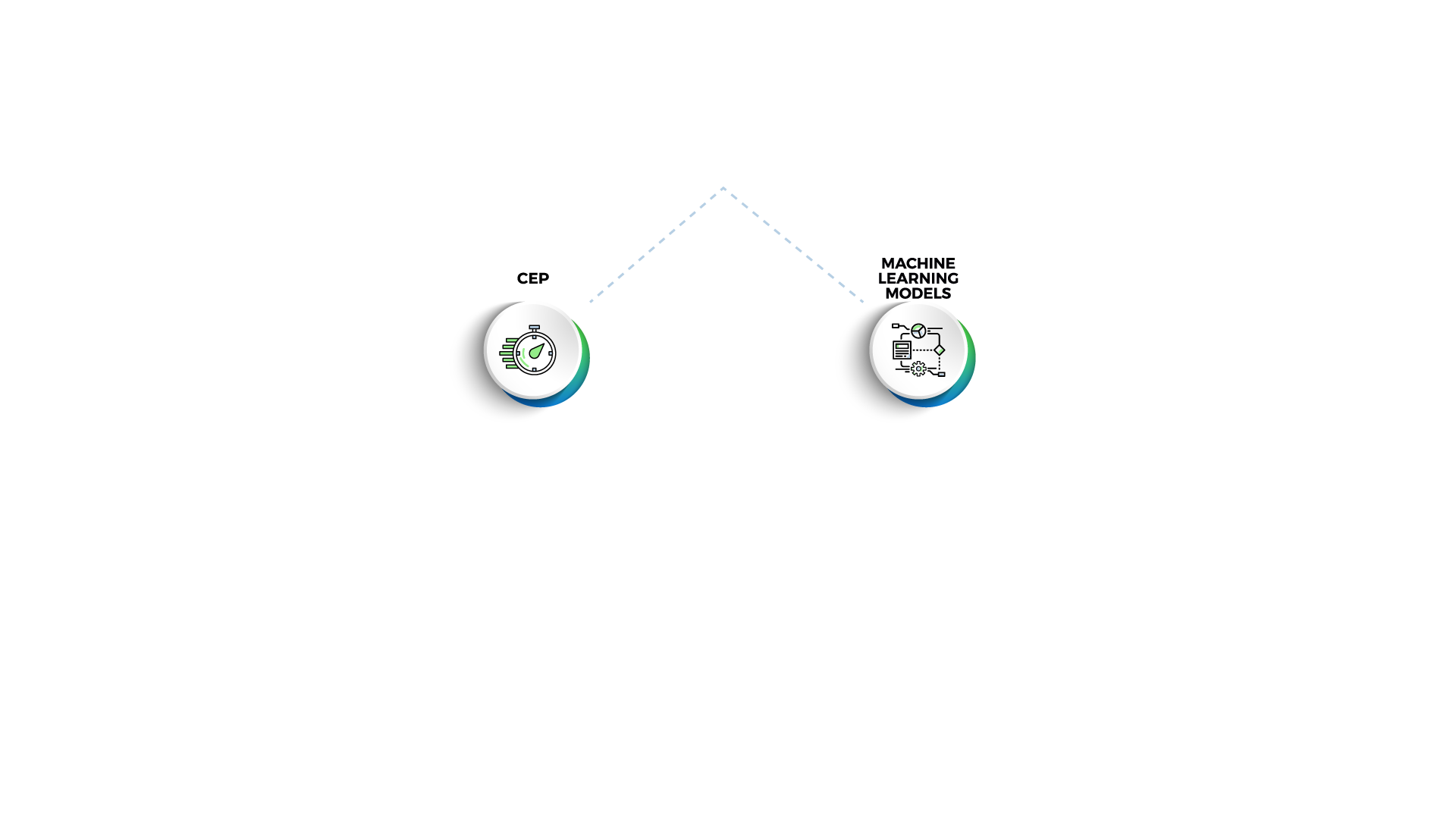
Saga d.o.o. Belgrade
Member of New Frontier Group
64a Zorana Đinđića Blvd.
11070 Beograd | SERBIA
saga.rs
selecta@saga.rs